Why is the weighted mean not included as an explanation for handling outliers?
Since a weighted mean considers how representative each data point is, outliers can be assigned lower weights. This reduces their influence on the calculated mean, leading to a more accurate representation of the central tendency.
Hey Graziano,
Good to hear from you!
How would you determine what weights is fair to assign to the outliers, please?
Best,
ned
Hey Ned, first of all I think there's no universal definition of 'fair' weights. The best approach probably depends on the nature of the data, the severity of outliers, and the goals of the data analysis. If you have information about the quality or certainty of the data points, assign lower weights to those we suspect may be less reliable (and potentially outliers). In some cases, an outlier might be a valid data point but irrelevant to the specific analysis, so we can downweight them (e.g. trimming, winsorizing etc... ) to focus on the more typical values. It's often worth experimenting with different weighting techniques to see how they impact the weighted mean and its interpretation. For example, using robust statistical methods like influence functions to mathematically determine weights based on how far a data point deviates from the center of the distribution, e.g. points further away (likely outliers), get progressively lower weights. Also, you can try the distance from the median. Methods like Huber loss weighting and Tukey's biweight can both help, depending on the cases. https://en.wikipedia.org/wiki/Robust_statistics
Submit an answer
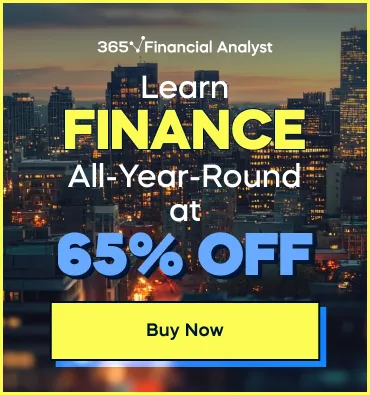